CS Colloquium: Theodore Papamarkou
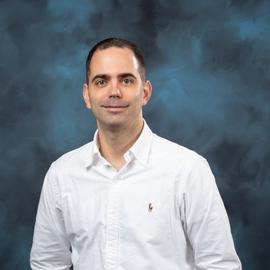
Speaker: Theodore Papamarkou
Date: Monday, September 26, 2022
Time: 3:30 - 4:30 pm
Location: HFH 1132
Host: Nina Mialone
Title: The premise of approximate MCMC in Bayesian deep learning
Abstract:
One of my primary research projects focuses on the development of approximate Markov chain Monte Carlo (MCMC) methods for Bayesian deep learning. Such methods are motivated by the problem of quantifying the uncertainty of predictions made by Bayesian neural networks.
Several challenges arise from sampling the parameter posterior of a neural network via MCMC, culminating to lack of convergence to the parameter posterior. Despite the lack of convergence, the approximate predictive posterior distribution contains valuable information (Papamarkou et al, 2022).
One step towards scaling MCMC methods to sample neural network parameters is based on evaluating the target density of a neural network on a subset (minibatch) of the data.
By analogy to sampling data batches from a big dataset, I propose to sample subgroups of parameters from the neural network parameter space (Papamarkou, 2022). While minibatch MCMC induces an approximation to the target density, parameter subgrouping can be carried out via blocked Gibbs sampling without introducing an additional approximation.
I will initially provide an overview of the developments for this project. Subsequently, I will outline plans for future computational and theoretical work that emerges from the proposal to sample each parameter subgroup separately.
Bio:
Theodore Papamarkou works as a reader in the mathematics of data science at The University of Manchester and is appointed as an adjunct professor at the University of Tennessee. Prior to his current role, Theodore held the positions of strategic hire in artificial intelligence at the Oak Ridge National Laboratory, and of assistant professor at the University of Glasgow.
In early stages of his career, he worked as a post-doctoral researcher at the University of Warwick, at University College London, and at the University of Cambridge. Theodore's research spans from Bayesian deep learning to mathematics of data science. By conducting research in these areas, he is interested in addressing questions related to uncertainty quantification for deep learning, and to approximate inference with big data or with high-dimensional models. In Bayesian deep learning, Theodore's two main research projects pertain to approximate Markov chain Monte Carlo (MCMC) and to neural network Gaussian processes (NNGPs). More details about his research activities and publications can be found here.