The UC Santa Barbara Computer Science Department hosts an annual summit.cs event, uniting undergraduate and graduate students, faculty, alumni, and industry partners in a program of sharing ideas and making connections. The 2023 event included Capstone presentations and awards, as well as a distinguished lecture by David Fenke, an American entrepreneur, businessman, and technical advisor. Fenke currently serves on the UCSB College of Engineering Dean’s Cabinet and has been a trustee of the UC Santa Barbara Foundation since 2017.
Watch summit.cs 2023 here.
2024 Agenda
12:00 - 1:00 PM : Lunch and Poster Session
1:00 PM : Opening Remarks
1:15 - 2:15 PM : 2024 Distinguished Lecture
2:30 - 5:00 PM : Capstone Presentations and Awards
5:00 - 6:00 PM : Reception & Awards
Sharing Ideas and Making Connections
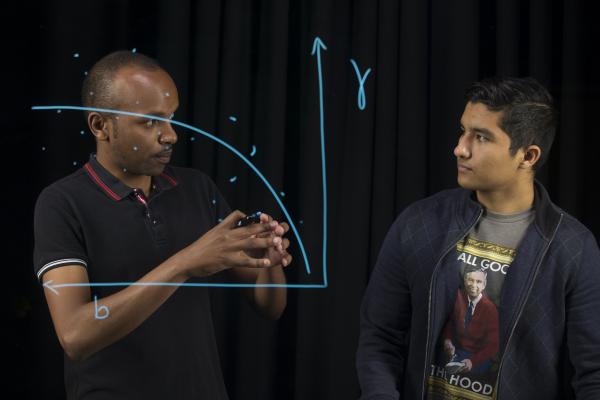
Undergrad Capstone
Teaming up with industry leaders, students worked on projects addressing a wide range of topics.
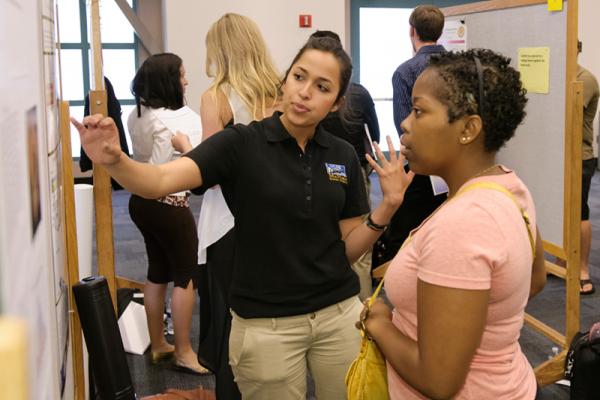
Poster Session
This is a chance to interact with the people behind the work. Each of the undergraduate capstone teams, along with a collection of undergraduate researchers, will be available to explain their ongoing work (with the help of a poster), and in some cases even provide demonstrations of their creations.
summit.cs 2023 Winners
First Place: TRANQUILITEA (partner: WellHealth)
Second Place: FAT STACKS (partner: Allthenticate)
Third Place: OVERSEA (partner: NavSea)
2023 Distinguished Lecture
David Henke '78
Computer Science Learnings for 45 years
Abstract:
David Henke is a 1978 graduate of UCSB in mathematics. There was no Computer Science major in 1978. He would like to share learnings from his career, including successes, failures, and challenges. Henke's axioms describe most of those learnings. Also, he would like to talk about future trends in computing and share any advice for the Computer Science engineers jumping into the fray.
Bio:
David Henke is an American entrepreneur, businessman, and technical advisor. He served as the senior vice president of engineering and operations for LinkedIn, a professional social networking website, from 2009 to 2013. Prior to LinkedIn, Henke was the SVP of engineering and operations at Yahoo for over four years. He has worked at AltaVista, Silicon Graphics, Hewlett Packard, and co-founded two successful startups, CAE Systems (acquired) and TeamOne Systems (acquired).
Mr. Henke received his bachelor’s degree in mathematics from UCSB in 1978, graduating with highest honors. He currently serves on the UCSB College of Engineering Dean’s Cabinet and is a trustee of the UC Santa Barbara Foundation since 2017.
Capstone & Event Sponsors